FDA’s AI White Paper: To Be or Not to Be, That is the Question
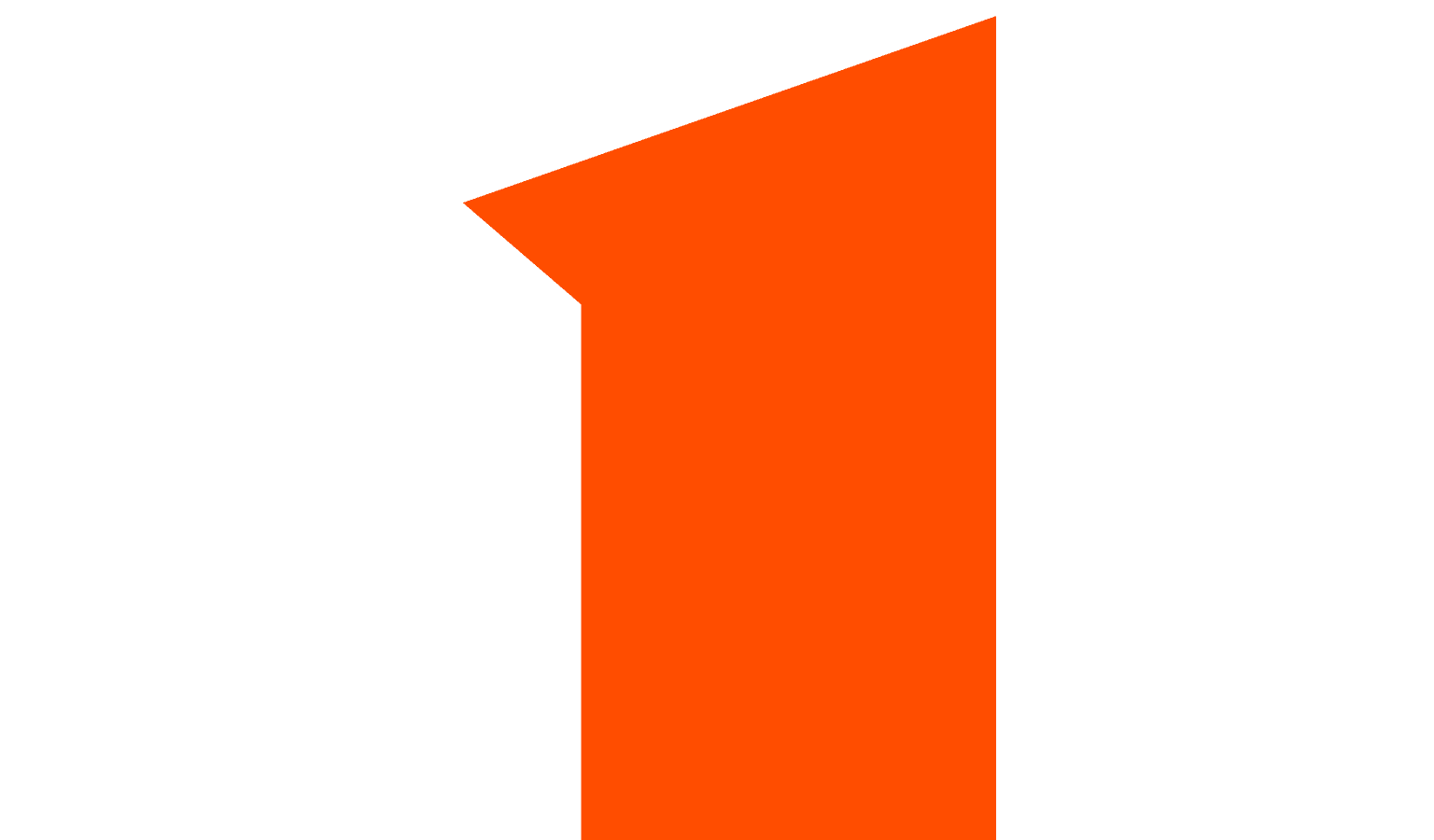
Key Points
- FDA has released a white paper outlining a potential regulatory framework for software as a medical device (SaMD) that leverages artificial intelligence (AI) or machine learning (ML).
- The white paper distinguishes three types of modifications to AI/ML-based SaMD, and describes how these types of changes might fit within the framework for evaluation of device modifications.
- FDA identifies four principles for AI/ML “learning algorithms,” including the use of an algorithm change protocol that might serve as an alternative to the nascent “Pre-Certification” pilot.
On April 2, the U.S. Food and Drug Administration (FDA) published a long-awaited exploratory white paper proposing a framework for regulating artificial intelligence/machine learning (AI/ML)-based software as a medical device (SaMD).1 Stakeholders are invited to comment on the discussion paper through June 3, 2019, at www.regulations.gov, Docket No. FDA-2019-N-1185.
Background and Working Definitions
FDA’s white paper highlights the challenges that AI/ML-based software poses to the traditional medical device regulatory framework. As FDA has acknowledged, AI products with algorithms that continually adapt based on new data are not well suited to the current regulatory paradigm, under which significant software modifications require a new premarket submission prior to marketing. Through the application of learning algorithms, SaMD may undergo rapid, if not constant, change. Perhaps the first challenge, however, is definitional: how to draw boundaries around what does and does not constitute AI/ML. For purposes of the paper, FDA describes “AI, and specifically ML, [as] techniques used to design and train software algorithms to learn from and act on data. These AI/ML-based software, when intended to treat, diagnose, cure, mitigate, or prevent disease or other conditions, are medical devices under the Food, Drug and Cosmetic Act, and called [SaMD] by FDA and the International Medical Device Regulators Forum (IMDRF).” The IMDRF takes a risk-based approach to categorizing SaMD based on intended use (similar to FDA’s traditional risk-based regulatory approaches), and identifies two main factors:
- Significance of information provided by the SaMD to the healthcare decision (e.g., to treat or diagnose, drive clinical management or inform clinical management).
- State of healthcare situation or condition (e.g., critical, serious or non-serious).
FDA identifies another variable applicable to AI/ML, apart from patient risk: a spectrum of dynamism ranging from “locked” to continuous learning. A locked algorithm provides the same result each time the same input is provided. FDA has recently approved several medical devices that rely on locked algorithms, such as a device used to detect diabetic retinopathy, and a device designed to alert providers of a potential stroke in patients. Continuously learning algorithms, on the other hand, are adaptive, changing their behavior using a defined learning process: “The algorithm adaptation or changes are implemented such that for a given set of inputs, the output may be different before and after the changes are implemented.” To our knowledge, FDA has not yet cleared or approved a device that employs an unlocked, continuous learning algorithm.
FDA also outlines possible modifications to an AI/ML-based SaMD. Although the agency recognizes that some modifications may not require premarket review under the current system, FDA anticipates that many modifications will involve “algorithm architecture modifications and re-training with new data sets,” which would be subject to premarket review. The white paper outlines three broad categories of modifications:
- Performance – clinical and analytical.
- Inputs – used by the algorithm and their clinical association to the SaMD output.
- Intended use – of the SaMD based on the risk categorization framework established by the IMDRF.
TPLC Approach
In section IV of the white paper, FDA gets to the meat of its regulatory proposal. FDA proposes a Total Product Life Cycle (TPLC) approach to modifications to AI/ML-based SaMD, based on several regulatory concepts: the IMDRF risk categorization principles, FDA’s risk-benefit framework, risk management principles in FDA’s Software Modifications guidance2 and the quality and organizational excellence-based TPLC approach FDA envisions for the Digital Health Software Precertification (“Pre-Cert”) Program. FDA admits that the innovative approach it proposes may require additional statutory authority (which may also be true for the software Pre-Cert program). FDA identifies four general principles that the agency believes appropriately balance benefits and risks, while providing access to safe and effective AI/ML-based SaMD:
- “Establish clear expectations on quality systems and good ML practices (GMLP).
- Conduct premarket review for those SaMD that require premarket submission to demonstrate reasonable assurance of safety and effectiveness and establish clear expectations for manufacturers of AI/ML-based SaMD to continually manage patient risks throughout the lifecycle.
- Expect manufacturers to monitor the AI/ML device and incorporate a risk management approach and other approaches outlined in [FDA’s Software Modifications Guidance] in development, validation, and execution of the algorithm changes (SaMD Pre-Specifications and Algorithm Change Protocol).
- Enable increased transparency to users and FDA using postmarket real-world performance reporting for maintaining continued assurance of safety and effectiveness.”
Good machine learning practices (GMLP)
The first principle, GMLP, reflects the agency’s expectations that medical device manufacturers have an established quality system that conforms to appropriate standards and regulation. Specific to AI/ML-based SaMD, FDA proposes relying on the Software Pre-Cert principles of culture of quality and organizational excellence for developers, and on its SaMD Clinical Evaluation Guidance for demonstrating analytical and clinical validation. FDA provides examples of GMLP considerations applicable to SaMD, including:
- “Relevance of available data to the clinical problem and current clinical practice
- Data acquired in a consistent, clinically relevant and generalizable manner that aligns with the SaMD’s intended use and modification plans
- Appropriate separation between training, tuning, and test datasets and
- Appropriate level of transparency (clarity) of the output and the algorithm aimed at users.”
Each of these considerations aligns in some way with the white paper published by the Xavier Health CLS (Continuous Learning Systems) Working Team, comprised of FDA officials and industry professionals working with Xavier Health’s AI Initiative.3 The Xavier CLS considerations are more detailed and fleshed out than those that FDA identifies in its white paper, and it stands to reason that FDA had the Xavier CLS considerations in mind.
Initial Premarket Assurance of Safety and Effectiveness
FDA does not address any unique aspects to the initial submission and clearance or approval of AI/ML-based SaMD. The white paper does, however, propose a framework for modifications to AI/ML-based SaMD that relies on the concept of a “predetermined change control plan,” which would be submitted during the initial premarket review of the device. The plan would include the types of anticipated modifications, “SaMD Pre-Specifications” (SPS), and the associated methodology, “Algorithm Change Protocol” (ACP), that would be used to implement the changes in a controlled manner designed to minimize patient risk. FDA notes that the extent to which SPS and ACP pre-approval would be appropriate to support future modifications depends on various factors, including the types of modifications (particularly as they relate to intended use) and their potential to introduce risks to patients. Notably, this approach is impliedly distinct from the Pre-Cert pilot, although by leveraging the existing de novo (and other) review pathways, the initial Pre-Cert pilot program may end up operating in a very similar manner to the proposed use of an ACP through a traditional premarket review pathway.
Approach for Modifications after Initial Review with an Established SPS and ACP
FDA indicates that many software modifications inherent to AI/ML-based SaMD would require a new premarket submission prior to marketing under the traditional FDA regulatory paradigm. The white paper proposes that if an AI/ML-based SaMD has an approved SPS and ACP, and modifications are within the bounds of both the SPS and ACP, manufacturers would simply document the change in the appropriate records and file for reference. If, on the other hand, the modification is beyond the approved SPS and ACP (but supports the same intended use), FDA proposes conducting a “focused review” to refine the SPS and ACP. If the modifications would result in a new intended use, they would be subject to premarket review.
Transparency and Real-World Performance Monitoring of AI/ML-Based SaMD
FDA expects manufacturers to commit to the principles of transparency and real-world performance monitoring, which the agency notes could be achieved through a variety of mechanisms. Transparency could be demonstrated by, for example, updates to FDA and collaborators; ensuring that labeling changes accurately describe modifications; updating the specifications or compatibility of impacted supporting devices, components or accessories; and establishing procedures to notify users of updates. For performance monitoring, FDA cites programs that are currently used or under pilot, like adding to an annual report, Case for Quality activities or real-world performance analytics pursuant to the Software Pre-Cert Program. FDA proposes leveraging pilot programs like Pre-Cert and Case for Quality, noting that involvement in these programs may impact the reporting type and frequency, given FDA’s particular insight into the manufacturer’s organization.
Questions and Considerations
The white paper provides a constructive starting point for evaluating the unique regulatory issues raised by AI/ML. Up to this point, commentary around the regulation of SaMD (and what software functionalities should be regulated as a medical device) has focused on the speed of software innovation, without necessarily distinguishing between rapid iteration (i.e., frequent version updates) and continuous learning. At the same time, commentary around digital health regulation generally has begun to distinguish between transparent algorithms and “black box” algorithms. “Black box” typically implies that an algorithm can be described and is predictable, but is treated by the sponsor as proprietary or is otherwise too complex to be understood by a user. This white paper initiates a more specific focus on the challenges presented by continuous learning algorithms that are beyond “black box,” and produce outcomes that might be unknowable to the sponsor. However, many of the concepts that FDA proposes have potential SaMD applications beyond the narrower context of continuous learning AI/ML, and it is not clear that these concepts are feasible for true continuous learning algorithms.
What actually constitutes AI or ML?
The white paper’s working definitions of AI and ML focus on software algorithms trained to “learn.” FDA contrasts a continuous learning algorithm (or adaptive algorithm), from a locked algorithm, which provides the same result each time based on a fixed function. A locked algorithm, therefore, may have the ability to learn, but it is not actively learning while deployed; stated another way, it is not applying AI or ML while marketed. If a SaMD presented for premarket review is locked, then the device is static, and whatever its complexity, it is not employing AI or ML in the marketed device. Thus, it is important to recognize that this white paper is proposing approaches that would facilitate the validation and implementation of AI/ML-generated advancements of SaMD, but it does not contemplate the deployment of adaptive AI/ML in marketed SaMD. In that sense, AI/ML remains a development tool, but is not itself functioning as SaMD.
Potential application of change protocols
The white paper primarily considers AI/ML in the context of changes to an already cleared or approved SaMD. As noted above, FDA proposes to rely on an Algorithm Change Protocol, which would identify methods that a manufacturer has in place to achieve and appropriately control the risks of the anticipated types of modifications to the algorithm. This proposal is consistent with approaches that FDA is taking in other contexts, such as next generation sequencing (NGS) tests for tumor profiling.4 Under FDA’s three-tiered approach for NGS tumor profiling tests, “test developers will be able to report additional variants of the same type post-market within the existing analytically validated genes in the panel, for claims consistent with the clinical criteria established in the original submission, without an additional FDA submission.” In addition to adding variants within a single level, biomarkers with potential clinical significance (level 3) may be able to graduate to be considered biomarkers with evidence of clinical significance (level 2) with sufficient data—but without additional FDA clearance. Similarly, FDA exempted certain genetic health risk tests from premarket review, provided the manufacturers undergo a one-time FDA review.5
The standards for device change that require premarket review are dictated in regulations.6 While those standards are famously subjective, the underlying criteria can be interpreted via guidance but can only be changed via regulation. The application of change protocols in an original submission, however, would leverage FDA’s statutory authority to define what constitutes the device at the time of classification and clearance/approval. The white paper’s description for the change protocols actually seems more feasible for SaMD with complex but locked algorithms, than for truly adaptive AI/ML-powered algorithms. It is unclear how developers would be able to meet these expectations, as the examples FDA identifies in the appendix relate to validating an enhancement that was developed via AI/ML, but which is then only added subsequently (either subject to a new submission or based on validation and documentation under the change protocol).
AI/ML for device-exempt functions
It is worth noting that this white paper addresses AI/ML used for SaMD. In many cases, AI/ML is being employed for patient engagement, clinician and provider support tools, and other health monitoring and analysis functions that do not constitute medical devices. For example, AI/ML can support clinical decision support tools and other software functionalities that are statutorily exempt from the medical device definition.7 This white paper does not alter those statutory exemptions or the underlying medical device definition. In circumstances in which AI/ML is being employed to support a software function that does not constitute SaMD, however, but its sponsor is contemplating a future version of the software that would function as a medical device, it would be advisable to consider FDA’s suggestions concerning change protocols and GMLPs, and implement to the extent possible. Doing so will likely facilitate the future preparation of a device marketing application.
How does the white paper relate to the Pre-Cert Program?
The Pre-Cert working model 1.0 acknowledges that it has not settled on a specific approach to modifications, and will follow the existing software modifications guidance under the current version. The white paper’s proposal, while not yet even in the form of a draft guidance, should be understood as an alternative approach for SaMD that employs AI/ML. Given that FDA already uses a somewhat similar approach in the context of next-generation sequencing tests, it is conceivable that the agency could informally apply some of the concepts in this white paper for current submissions. For example, without having issued specific guidance, FDA has allowed sponsors of NGS tests to add biomarkers to their test panels without an additional clearance or approval, after the biomarkers have been validated. As FDA has acknowledged, and much like algorithm modifications in the AI/ML space, these changes would normally constitute a modification requiring a new submission and authorization before marketing. Developers of SaMD with AL/ML should consider proposing some of the concepts articulated in this white paper, despite its conceptual status.
Contact Information
If you have any questions concerning this alert, please contact:
Nathan Brown |
Christin Carey |
Howard Sklamberg |
Marlee Gallant |
1 Proposed Regulatory Framework for Modifications to Artificial Intelligence/Machine Learning (AL/ML-Based Software as a Medical Device (SaMD), Discussion Paper and Request for Feedback (April 2019)), https://www.fda.gov/downloads/MedicalDevices/DigitalHealth/SoftwareasaMedicalDevice/UCM635052.pdf.
2 FDA Guidance, Deciding When to Submit a 510(k) for a Software Change to an Existing Device (Oct. 2017), https://www.fda.gov/downloads/medicaldevices/deviceregulationandguidance/guidancedocuments/ucm514737.pdf.
3 Xavier University Exhibit, Perspectives and Best Practices for Artificial Intelligence and Continuously Learning Systems in Healthcare (Summer 2018) https://www.exhibit.xavier.edu/cgi/viewcontent.cgi?article=1024&context=health_services_administration_faculty.
4 See https://www.fda.gov/downloads/medicaldevices/productsandmedicalprocedures/invitrodiagnostics/ucm584603.pdf.
5 FDA, Final Order; Medical Devices; Exemptions from Premarket Notification: Class II Devices, 83 Fed. Reg. 25,910 (June 5, 2018), https://www.federalregister.gov/documents/2018/06/05/2018-11879/medical-devices-exemptions-from-premarket-notification-class-ii-devices.
6 See 21 C.F.R. § 814.39 (for a device approved via a PMA, a new submission is required when making any change affecting the safety or effectiveness of the device); id. § 807.81(a)(3) (for a device cleared via 510(k), a new submission is required when making a change that could significantly affect the safety or effectiveness of the device, or major changes or modifications in the intended use of the device).
7 See 21 U.S.C. § 360j(o) (added by Section 3060 of the 21stCentury Cures Act of 2016).